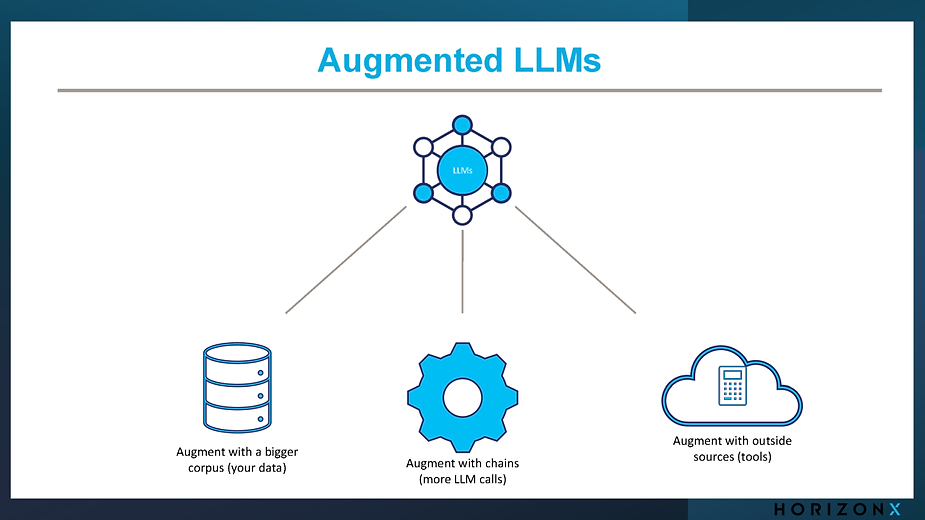
A new horizon is rising in the dynamic digital transformation landscape, where technology continually shapes how we operate. I find myself at the forefront of this evolution at HorizonX, a tech innovation hub dedicated to delivering cutting-edge digital solutions with cloud and data at the core.
We are currently navigating the era of Generation AI, characterised by the seamless integration of Neural Networks, which places us at the threshold of an exciting new chapter in technical evolution. It prompts us to ask:
What's next?
The answer lies in Augmented Language Models (LLMs), a captivating frontier that promises to redefine how we interact with technology and data.
This article will explore why LLMs are the logical progression in our digital transformation journey, particularly when we focus on innovative techniques like Retrieval and LangChain. But first, let's lay the groundwork by understanding the role of language models in today's tech landscape.
Language Models: A Primer
Despite the remarkable advancements in language models like GPT-4, LlaMA, BARD, and Falcon, they still exhibit significant limitations. For instance, their knowledge cutoff restricts them from having up-to-date information on world events. Furthermore, while they excel in language understanding, basic reasoning, and code comprehension, they cannot interact with the world autonomously. One could compare them to brilliant high school students with immense raw potential but need tools and data to function optimally.
Comparative Analysis
When we discuss the landscape of language models, it's crucial to acknowledge the groundbreaking advancements represented by models like GPT-4 - in language models, boasting impressive capabilities in understanding and generating human language. However, to fully appreciate the potential of Augmented Language Models (LLMs), it's essential to understand the distinctions and innovations they bring to the table.
Augmented LLMs (Augmented Language Models) represent a paradigm shift in the evolution of language models. Unlike conventional models like GPT-4, LLMs go beyond the boundaries of raw language understanding. They are designed with a specific focus on practical, real-world applications, and this distinction is what sets them apart.
One of the key differentiators is incorporating advanced techniques such as Retrieval and LangChain within LLMs. These techniques act as bridges, connecting the model's vast knowledge and language proficiency to tangible real-world scenarios.
The Power of Context
A key to optimising LLMs lies in providing them with the right context. Think of it as giving them a "cheat sheet" during an exam. To maximise their performance, we must ensure our context is rich, updated, and relevant. This is where techniques like Retrieval come into play.
Retrieval: The First Step
LLMs are equipped with a powerful capability known as Retrieval. In simple terms, Retrieval is a technique where LLMs are augmented with an external data corpus they can search to answer queries. It's somewhat akin to a high-powered search engine. The challenge lies in refining this search. Traditional metrics might look at keyword frequency or document relevance. However, with advancements in AI, there's potential for more nuanced search capabilities.
For instance, an LLM augmented with Retrieval could filter through medical journals to provide the latest findings on a specific disease or treatment method in the healthcare sector.
LangChain: An Advanced Technique
LangChain takes the concept of information extraction a step further. Instead of merely extracting raw data, LLMs can use LangChain to retrieve summaries or condensed versions of information. LangChain is a method where multiple LLMs are linked together. Instead of just extracting raw data, an LLM could retrieve summaries or even summaries of summaries, leading to more concise and relevant information.
This could mean that a business analyst looking for market trends might receive a distilled version of multiple reports curated to offer the most pertinent insights. It's an iterative chain of models, each enhancing the output of the previous.
The Future: Integration with External Tools
Many wonder, "Why not just use Google?" The distinction lies in the intricacies. While search engines like Google provide vast information, LLMs offer a refined, context-aware response tailored to user queries, particularly when augmented with tools like Retrieval and LangChain.
Consider a system where a user's question is transformed into an SQL query by the LLM. The resulting data from a live database becomes part of the model's context, yielding precise and relevant answers. This is the power and potential that LLMs bring to the table.
Conclusion
At the exciting intersection of AI and digital transformation that our dedicated teams at HorizonX are navigating, Augmented Language Models stand out as a beacon of potential. By integrating techniques like Retrieval and LangChain and comparing their strengths with existing models, we can tap into their unparalleled capabilities and redefine our problem-solving and data analysis approach. Our journey into the world of tech innovation, driven by HorizonX, is just beginning, and with LLMs, the future looks brighter than ever.
A new horizon is rising in the dynamic digital transformation landscape, where technology continually shapes how we operate. I find myself at the forefront of this evolution at HorizonX, a tech innovation hub dedicated to delivering cutting-edge digital solutions with cloud and data at the core.
We are currently navigating the era of Generation AI, characterised by the seamless integration of Neural Networks, which places us at the threshold of an exciting new chapter in technical evolution. It prompts us to ask:
What's next?
The answer lies in Augmented Language Models (LLMs), a captivating frontier that promises to redefine how we interact with technology and data.
This article will explore why LLMs are the logical progression in our digital transformation journey, particularly when we focus on innovative techniques like Retrieval and LangChain. But first, let's lay the groundwork by understanding the role of language models in today's tech landscape.
Language Models: A Primer
Despite the remarkable advancements in language models like GPT-4, LlaMA, BARD, and Falcon, they still exhibit significant limitations. For instance, their knowledge cutoff restricts them from having up-to-date information on world events. Furthermore, while they excel in language understanding, basic reasoning, and code comprehension, they cannot interact with the world autonomously. One could compare them to brilliant high school students with immense raw potential but need tools and data to function optimally.
Comparative Analysis
When we discuss the landscape of language models, it's crucial to acknowledge the groundbreaking advancements represented by models like GPT-4 - in language models, boasting impressive capabilities in understanding and generating human language. However, to fully appreciate the potential of Augmented Language Models (LLMs), it's essential to understand the distinctions and innovations they bring to the table.
Augmented LLMs (Augmented Language Models) represent a paradigm shift in the evolution of language models. Unlike conventional models like GPT-4, LLMs go beyond the boundaries of raw language understanding. They are designed with a specific focus on practical, real-world applications, and this distinction is what sets them apart.
One of the key differentiators is incorporating advanced techniques such as Retrieval and LangChain within LLMs. These techniques act as bridges, connecting the model's vast knowledge and language proficiency to tangible real-world scenarios.
The Power of Context
A key to optimising LLMs lies in providing them with the right context. Think of it as giving them a "cheat sheet" during an exam. To maximise their performance, we must ensure our context is rich, updated, and relevant. This is where techniques like Retrieval come into play.
Retrieval: The First Step
LLMs are equipped with a powerful capability known as Retrieval. In simple terms, Retrieval is a technique where LLMs are augmented with an external data corpus they can search to answer queries. It's somewhat akin to a high-powered search engine. The challenge lies in refining this search. Traditional metrics might look at keyword frequency or document relevance. However, with advancements in AI, there's potential for more nuanced search capabilities.
For instance, an LLM augmented with Retrieval could filter through medical journals to provide the latest findings on a specific disease or treatment method in the healthcare sector.
LangChain: An Advanced Technique
LangChain takes the concept of information extraction a step further. Instead of merely extracting raw data, LLMs can use LangChain to retrieve summaries or condensed versions of information. LangChain is a method where multiple LLMs are linked together. Instead of just extracting raw data, an LLM could retrieve summaries or even summaries of summaries, leading to more concise and relevant information.
This could mean that a business analyst looking for market trends might receive a distilled version of multiple reports curated to offer the most pertinent insights. It's an iterative chain of models, each enhancing the output of the previous.
The Future: Integration with External Tools
Many wonder, "Why not just use Google?" The distinction lies in the intricacies. While search engines like Google provide vast information, LLMs offer a refined, context-aware response tailored to user queries, particularly when augmented with tools like Retrieval and LangChain.
Consider a system where a user's question is transformed into an SQL query by the LLM. The resulting data from a live database becomes part of the model's context, yielding precise and relevant answers. This is the power and potential that LLMs bring to the table.
Conclusion
At the exciting intersection of AI and digital transformation that our dedicated teams at HorizonX are navigating, Augmented Language Models stand out as a beacon of potential. By integrating techniques like Retrieval and LangChain and comparing their strengths with existing models, we can tap into their unparalleled capabilities and redefine our problem-solving and data analysis approach. Our journey into the world of tech innovation, driven by HorizonX, is just beginning, and with LLMs, the future looks brighter than ever.
Augmented Language Models: The Next Frontier in Digital Transformation
A new horizon is rising in the dynamic digital transformation landscape, where technology continually shapes how we operate. I find myself at the forefront of this evolution at HorizonX, a tech innovation hub dedicated to delivering cutting-edge digital solutions with cloud and data at the core.
We are currently navigating the era of Generation AI, characterised by the seamless integration of Neural Networks, which places us at the threshold of an exciting new chapter in technical evolution. It prompts us to ask:
What's next?
The answer lies in Augmented Language Models (LLMs), a captivating frontier that promises to redefine how we interact with technology and data.
This article will explore why LLMs are the logical progression in our digital transformation journey, particularly when we focus on innovative techniques like Retrieval and LangChain. But first, let's lay the groundwork by understanding the role of language models in today's tech landscape.
Language Models: A Primer
Despite the remarkable advancements in language models like GPT-4, LlaMA, BARD, and Falcon, they still exhibit significant limitations. For instance, their knowledge cutoff restricts them from having up-to-date information on world events. Furthermore, while they excel in language understanding, basic reasoning, and code comprehension, they cannot interact with the world autonomously. One could compare them to brilliant high school students with immense raw potential but need tools and data to function optimally.
Comparative Analysis
When we discuss the landscape of language models, it's crucial to acknowledge the groundbreaking advancements represented by models like GPT-4 - in language models, boasting impressive capabilities in understanding and generating human language. However, to fully appreciate the potential of Augmented Language Models (LLMs), it's essential to understand the distinctions and innovations they bring to the table.
Augmented LLMs (Augmented Language Models) represent a paradigm shift in the evolution of language models. Unlike conventional models like GPT-4, LLMs go beyond the boundaries of raw language understanding. They are designed with a specific focus on practical, real-world applications, and this distinction is what sets them apart.
One of the key differentiators is incorporating advanced techniques such as Retrieval and LangChain within LLMs. These techniques act as bridges, connecting the model's vast knowledge and language proficiency to tangible real-world scenarios.
The Power of Context
A key to optimising LLMs lies in providing them with the right context. Think of it as giving them a "cheat sheet" during an exam. To maximise their performance, we must ensure our context is rich, updated, and relevant. This is where techniques like Retrieval come into play.
Retrieval: The First Step
LLMs are equipped with a powerful capability known as Retrieval. In simple terms, Retrieval is a technique where LLMs are augmented with an external data corpus they can search to answer queries. It's somewhat akin to a high-powered search engine. The challenge lies in refining this search. Traditional metrics might look at keyword frequency or document relevance. However, with advancements in AI, there's potential for more nuanced search capabilities.
For instance, an LLM augmented with Retrieval could filter through medical journals to provide the latest findings on a specific disease or treatment method in the healthcare sector.
LangChain: An Advanced Technique
LangChain takes the concept of information extraction a step further. Instead of merely extracting raw data, LLMs can use LangChain to retrieve summaries or condensed versions of information. LangChain is a method where multiple LLMs are linked together. Instead of just extracting raw data, an LLM could retrieve summaries or even summaries of summaries, leading to more concise and relevant information.
This could mean that a business analyst looking for market trends might receive a distilled version of multiple reports curated to offer the most pertinent insights. It's an iterative chain of models, each enhancing the output of the previous.
The Future: Integration with External Tools
Many wonder, "Why not just use Google?" The distinction lies in the intricacies. While search engines like Google provide vast information, LLMs offer a refined, context-aware response tailored to user queries, particularly when augmented with tools like Retrieval and LangChain.
Consider a system where a user's question is transformed into an SQL query by the LLM. The resulting data from a live database becomes part of the model's context, yielding precise and relevant answers. This is the power and potential that LLMs bring to the table.
Conclusion
At the exciting intersection of AI and digital transformation that our dedicated teams at HorizonX are navigating, Augmented Language Models stand out as a beacon of potential. By integrating techniques like Retrieval and LangChain and comparing their strengths with existing models, we can tap into their unparalleled capabilities and redefine our problem-solving and data analysis approach. Our journey into the world of tech innovation, driven by HorizonX, is just beginning, and with LLMs, the future looks brighter than ever.
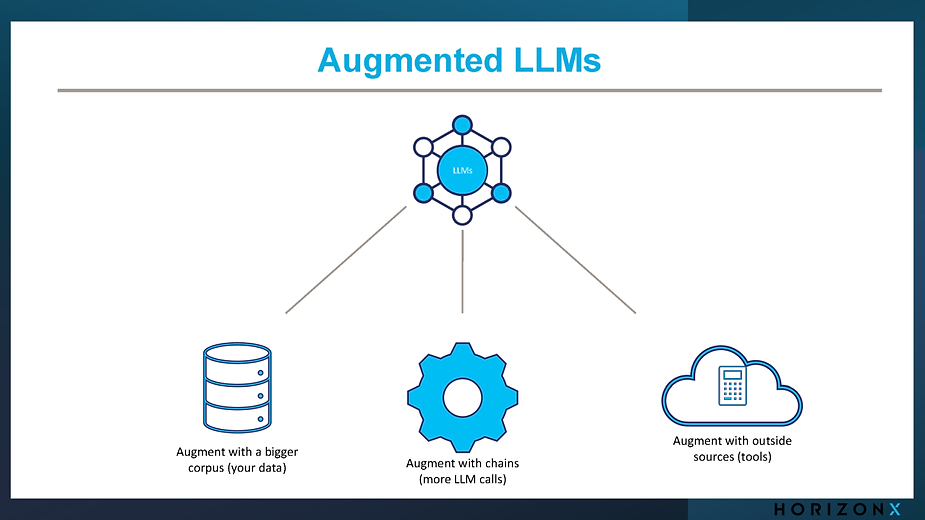
Download Checklist
Augmented Language Models: The Next Frontier in Digital Transformation
A new horizon is rising in the dynamic digital transformation landscape, where technology continually shapes how we operate. I find myself at the forefront of this evolution at HorizonX, a tech innovation hub dedicated to delivering cutting-edge digital solutions with cloud and data at the core.
We are currently navigating the era of Generation AI, characterised by the seamless integration of Neural Networks, which places us at the threshold of an exciting new chapter in technical evolution. It prompts us to ask:
What's next?
The answer lies in Augmented Language Models (LLMs), a captivating frontier that promises to redefine how we interact with technology and data.
This article will explore why LLMs are the logical progression in our digital transformation journey, particularly when we focus on innovative techniques like Retrieval and LangChain. But first, let's lay the groundwork by understanding the role of language models in today's tech landscape.
Language Models: A Primer
Despite the remarkable advancements in language models like GPT-4, LlaMA, BARD, and Falcon, they still exhibit significant limitations. For instance, their knowledge cutoff restricts them from having up-to-date information on world events. Furthermore, while they excel in language understanding, basic reasoning, and code comprehension, they cannot interact with the world autonomously. One could compare them to brilliant high school students with immense raw potential but need tools and data to function optimally.
Comparative Analysis
When we discuss the landscape of language models, it's crucial to acknowledge the groundbreaking advancements represented by models like GPT-4 - in language models, boasting impressive capabilities in understanding and generating human language. However, to fully appreciate the potential of Augmented Language Models (LLMs), it's essential to understand the distinctions and innovations they bring to the table.
Augmented LLMs (Augmented Language Models) represent a paradigm shift in the evolution of language models. Unlike conventional models like GPT-4, LLMs go beyond the boundaries of raw language understanding. They are designed with a specific focus on practical, real-world applications, and this distinction is what sets them apart.
One of the key differentiators is incorporating advanced techniques such as Retrieval and LangChain within LLMs. These techniques act as bridges, connecting the model's vast knowledge and language proficiency to tangible real-world scenarios.
The Power of Context
A key to optimising LLMs lies in providing them with the right context. Think of it as giving them a "cheat sheet" during an exam. To maximise their performance, we must ensure our context is rich, updated, and relevant. This is where techniques like Retrieval come into play.
Retrieval: The First Step
LLMs are equipped with a powerful capability known as Retrieval. In simple terms, Retrieval is a technique where LLMs are augmented with an external data corpus they can search to answer queries. It's somewhat akin to a high-powered search engine. The challenge lies in refining this search. Traditional metrics might look at keyword frequency or document relevance. However, with advancements in AI, there's potential for more nuanced search capabilities.
For instance, an LLM augmented with Retrieval could filter through medical journals to provide the latest findings on a specific disease or treatment method in the healthcare sector.
LangChain: An Advanced Technique
LangChain takes the concept of information extraction a step further. Instead of merely extracting raw data, LLMs can use LangChain to retrieve summaries or condensed versions of information. LangChain is a method where multiple LLMs are linked together. Instead of just extracting raw data, an LLM could retrieve summaries or even summaries of summaries, leading to more concise and relevant information.
This could mean that a business analyst looking for market trends might receive a distilled version of multiple reports curated to offer the most pertinent insights. It's an iterative chain of models, each enhancing the output of the previous.
The Future: Integration with External Tools
Many wonder, "Why not just use Google?" The distinction lies in the intricacies. While search engines like Google provide vast information, LLMs offer a refined, context-aware response tailored to user queries, particularly when augmented with tools like Retrieval and LangChain.
Consider a system where a user's question is transformed into an SQL query by the LLM. The resulting data from a live database becomes part of the model's context, yielding precise and relevant answers. This is the power and potential that LLMs bring to the table.
Conclusion
At the exciting intersection of AI and digital transformation that our dedicated teams at HorizonX are navigating, Augmented Language Models stand out as a beacon of potential. By integrating techniques like Retrieval and LangChain and comparing their strengths with existing models, we can tap into their unparalleled capabilities and redefine our problem-solving and data analysis approach. Our journey into the world of tech innovation, driven by HorizonX, is just beginning, and with LLMs, the future looks brighter than ever.
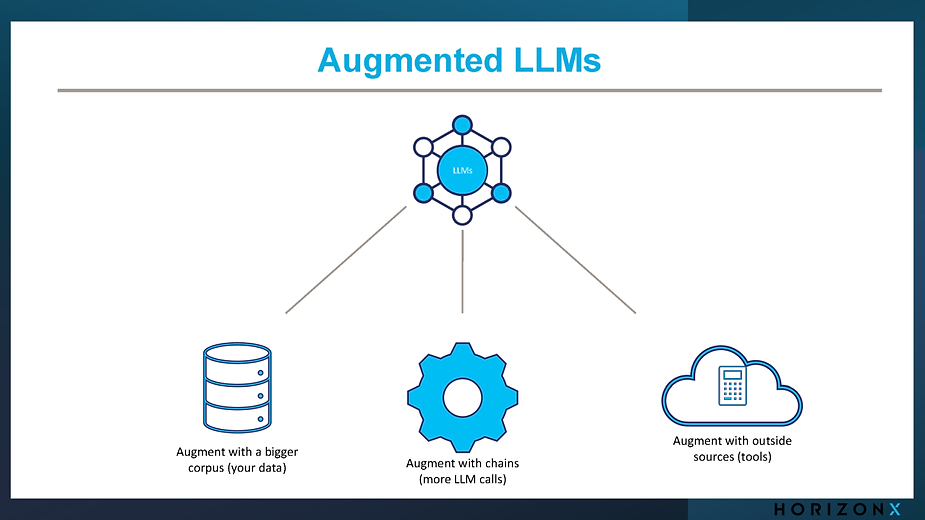
Download eBook

HorizonX Data Governance Checklist
Download our comprehensive Data Governance Checklist: to discover Identify gaps and assessing readiness.
Related Insights
Unlock new opportunities today.
Whether you have a question, a project in mind, or just want to discuss possibilities, we're here to help. Contact us today, and let’s turn your ideas into impactful solutions.